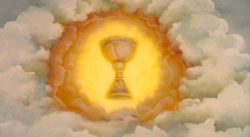
Last April a paper was published in an obscure journal that may have profound implications for the ME/CFS community. The paper was titled "Identifying Defining Aspects of Chronic Fatigue Syndrome via Unsupervised Machine Learning and Feature Selection" and it appeared in the International Journal of Machine Learning and Computing. Perhaps it is unfair to characterize this journal as obscure, as it is probably well known to computer science majors everywhere. It is obscure, however, to the IOM ... and to physicians, and to clinical researchers, and to pharma, and to anyone not in the computer science loop.
The reason this paper is so important, and why it can potentially put an end to the case definition war, is that the researchers have produced an instrument that not only will predict ME/CFS with greater accuracy than all existing case definitions, but can be used by anybody - physicians, researchers, people designing studies for pharma, the CDC, HHS, and even patients themselves.
How did they do it?
What the research team did was employ a computer algorithm normally used for data mining and clustering to sift through the answers on Jason's DePaul Symptom Questionnaire (DSQ). The DSQ contains 54 ME/CFS symptoms, which patients rank by frequency and severity. (These are the two parameters not included in the Fukuda definition, and which Lily Chu correctly insisted be included in any definition produced by the IOM.)
They then allowed the algorithm to crunch the data. Fifteen symptoms were prominent:
Modestly, the researchers proposed that their findings "hold promise for the development of an empirical CFS case definition as an accurate diagnostic tool." They suggest that "future work should focus on extending these findings to standardized criteria that can be easily implemented in a clinical setting."
The beauty of this elegant instrument is that by adjusting for symptoms that are prominent among some, but not all, people with ME/CFS, it can also be used to identify subgroups.
Without a doubt, other clinical features of this disease that are revealed by immune system, endocrine system, and nervous system tests would have to be added, as well as other objective, measurable abnormalities such as the two-day CPET. But so far, this "unsupervised machine learning and feature selection" tool is the best jumping-off point that has been developed.
Now, I believe, would be a good time for those seeking to devise an accurate case definition for ME/CFS to enter into the 21st century.
Read the full study HERE.
__________________
Citation: Samuel P. Watson, Amy S. Ruskin, Valerie Simonis, Leonard A. Jason, Madison Sunnquist, and Jacob D. Furst, "Identifying Defining Aspects of Chronic Fatigue Syndrome via Unsupervised Machine Learning and Feature Selection," International Journal of Machine Learning and Computing vol.4, no. 2, pp. 133-138, 2014.